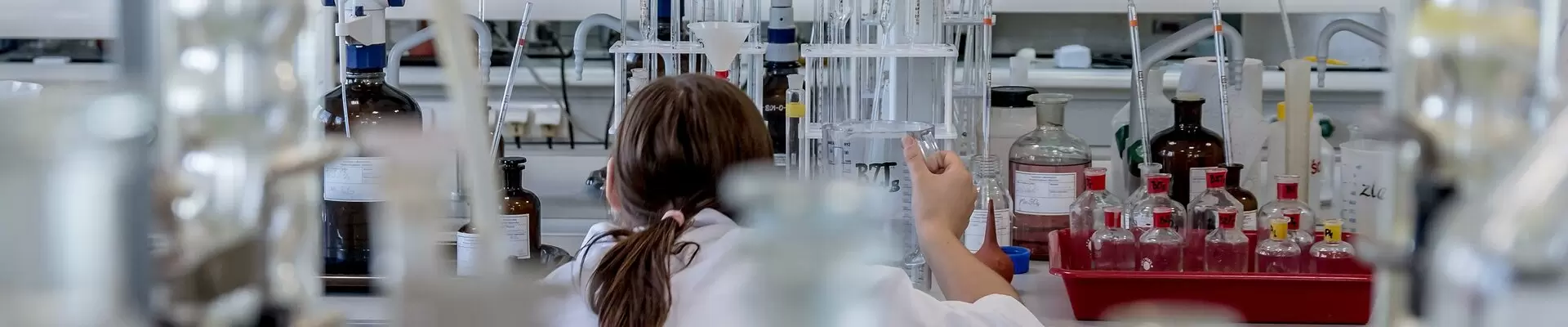
Laser Oximetry – Towards Real Time Personal Health Monitoring
With the advancement of wearable technology, personal health monitoring has become a reality. The availability of personal health monitoring provides users many possible customised health related programs, such as personal coaches for sport and fitness training, 24-hour health monitoring for the elderly, and advanced warnings for patients with hidden illness such as blood circulation and heart beat irregularities. A range of sensors and devices are needed to enable personal health monitoring, and optical pulse oximetry is one of them. Optical pulse oximetry has been commonly used in hospitals for clinical monitoring of heart rates and oxygen levels in blood. It utilises light source(s) to measure the photoplethysmograph (PPG) spectrum from the blood vessels. PPG spectrum carries a lot more information; for example, it could derive blood pressure, on-set of irregular heart rhythm. However, in order to be able to extract this information, the PPG spectrum has to be stable and robust over a long period of time. Presently, LED light sources are unable to do that because it's a broad, diffused light source leading to low signal. Therefore, instead of using LED as the light source, laser is a far better alternative, as it can penetrate soft tissue such as skin significantly deeper and therefore provide a much improved signal. By using lasers as sources producing superior SNR – laser oximetry, this project has the potential to improve personal health monitoring systems, providing real-time monitoring of health not only for elite athletes, fitness and sport participants, but also for long-term patients that require round-the-clock health monitoring.
Research Output
- Patent title: Organic laser for measurement. Patent No.: US 9,614,346 B2. Date of Patent: Apr. 4, 2017.
- K. L. Chan, G. X. Li and K. W. Cheah, Optical Material Express, 5, 497-502 (2015).
- G. Li, B. Choi, J. Xu, S. S. Bhowmick, K.P. Chun and G. L.H. Wong:
Efficient Shapelet Discovery for Time Series Classification, IEEE Transactions on Knowledge and Data Engineering (TKDE), accepted to appear, 2020. - G. Li, B. Choi, S. S. Bhowmick, G. L-H. Wong, K-P. Chun and S. Li.
"Visualet: Visualizing Shapelets for Time Series Classification."
Proc. of the 29th ACM International Conference on Information and Knowledge Management (CIKM '20), online, Oct 2020.
Demon video: