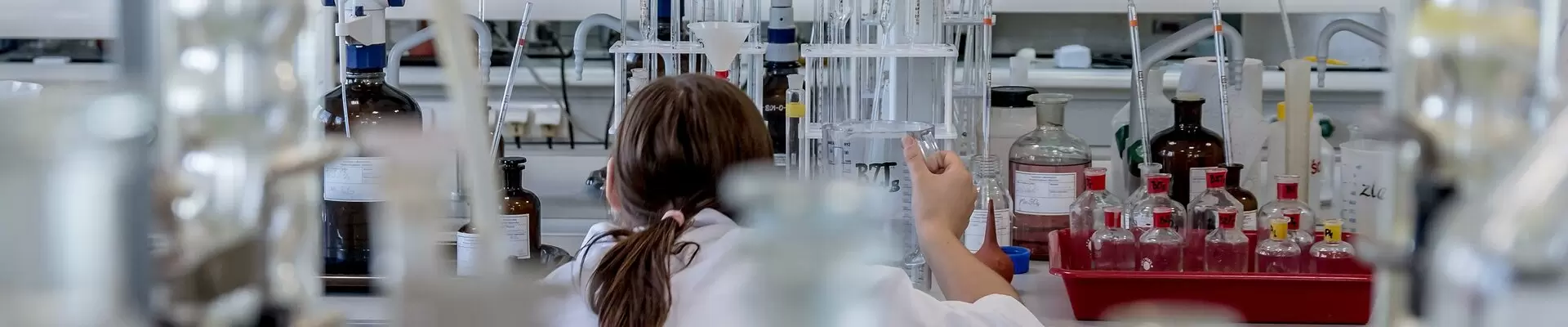
Interpretable Machine Learning for Predicting Individual Differences from Big Brain Data
The grand challenge of our time is to understand how “brains work and go wrong”. Advanced neuroimaging technologies, such as EEG and fMRI applied during resting states, and cognitive task processing have moved neuroscience research into the era of big data. This shifts the paradigm from the traditional hypothesis-based, group comparison approaches to an emerging and rapidly growing field of using Machine Learning tools for image processing, marker searching, disease diagnosis and for predicting individual differences from large-scale, multimodal neuroimaging data. Such data-driven approaches are highly promising for learning intricate latent relationships between brain and behaviour. Furthermore, the classification power of machine learning methods may help to translate large-scale brain imaging data into objective criteria for potential diagnoses and prognoses of disease in individuals. However, the data-learned relationships are usually opaque and the feature space often huge, making it difficult to interpret the results in terms of understanding how the brain works and what changes occur when it goes wrong.
This collaborative project aims to integrate and synergize existing expertise within HKBU to form an interdisciplinary team together with our international collaborators. We seek to build capacity for long-term development of big-data brain studies, in order to characterize and understand continuous spectra of cognitive behavioural differences between individuals. Researchers from advanced data analytics (pattern recognition and machine/deep learning), and dynamical network science (principle-based modelling) will closely work together with experts from cognitive neuroscience and differential psychology.
Models and understanding from the selected cognitive tasks within this project will build the foundation of a more comprehensive study of behaviours across many domains in the future. The project will contribute to elucidating a continuous spectra of multimodal features across individuals, with population-level priors used to predict risk of disorder for individuals at the extreme boundaries of the spectra. The research work and capacity built in this project will set a foundation for future projects to develop tools and applications to help educators or professionals conduct screenings to identify individuals at risk.
Figure 1. Schematics of the project.
Figure 2. Large-scale brain imaging data from Human Commectome Project (HCP) allowed us to identify the reading-related network and predict reading ability (from: Daniel Kristanto, Mianxin Liu, Xinyang Liu, Werner Sommer*, Changsong Zhou*, Predicting Reading Ability from Brain Anatomy and Function: From Areas to Connections, NeuroImage, 218, 116966 (2020)).
Research Outcomes:
- Mianxin Liu, Xinyang Liu, Andrea Hildebrandt and Changsong Zhou*, Individual cortical entropy profile: Test–retest reliability, predictive power for cognitive ability, and neuroanatomical foundation, Cerebral Cortex Communications. https://doi.org/10.1093/texcom/tgaa015
- Zedong Bi and Changsong Zhou, Understanding the computation of time using neural network models, Proc Natl Acad Sci USA, 117, 10530-10540 (2020). https://doi.org/10.1073/pnas.1921609117
- Xinyang Liu, Andrea Hildebrandt, Kristina Meyer, Werner Sommer, Changsong Zhou, Patterns of individual differences in fiber tract integrity of the face processing brain network support neurofunctional models, NeuroImage, 204, 116229 (2020). https://doi.org/10.1016/j.neuroimage.2019.116229
- Daniel Kristanto, Mianxin Liu, Xinyang Liu, Werner Sommer*, Changsong Zhou*, Predicting Reading Ability from Brain Anatomy and Function: From Areas to Connections, NeuroImage, 218, 116966 (2020). https://doi.org/10.1016/j.neuroimage.2020.116966
- Y. Zhao, Y.M. Cheung, X.G. You, Q.M. Peng, J.T. Peng, P.P. Yuan and Y.F. Shi, “Hyperspectral Image Classification via Spatial Window based Multi-view Intact Feature Learning”, IEEE Transactions on Geoscience and Remote Sensing, DOI:10.1109/TGRS.2020.3004858.
- X. Liu, Y.M. Cheung, Z.K. Hu, Y. He, B.E. Zhong and H.Y. Li, “Adversarial Tri-Fusion Hashing Network for Imbalanced Cross-Modal Retrieval”, IEEE Transactions on Emerging Topics in Computational Intelligence, DOI: 10.1109/TETCI.2020.3007143.
Principal Investigator
Co-Investigators (Non-Lab Members)

Prof. Andrea HILDEBRANDT (External)
University of Oldenburg