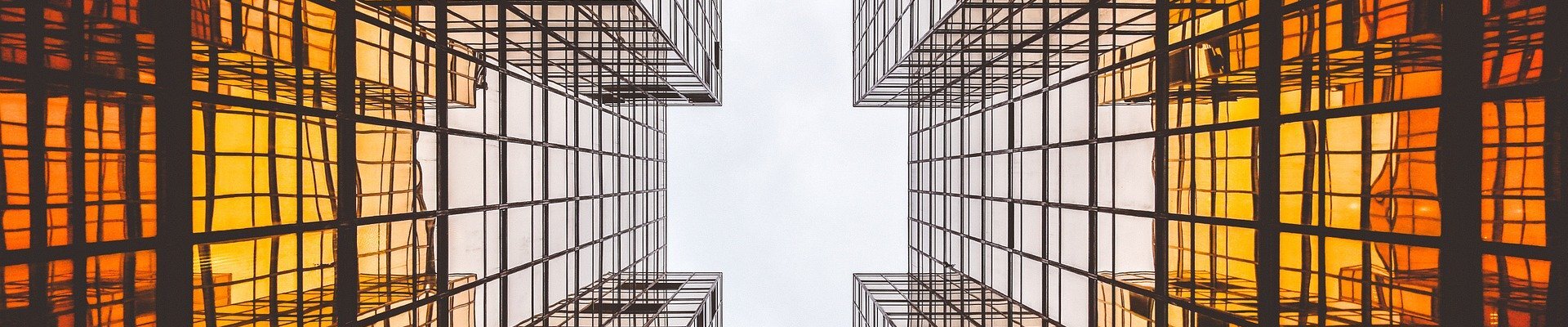
Statistical Learning for Heterogeneous Biomedical Data
02Apr
Speaker

Dr. Guanhua Chen
Assistant Professor
Biostatistics and Medical Informatics
University of Wisconsin-Madison
USA
Time
1430-1530, 02 Apr 2019
Venue
SCT909, Cha Chi Ming Science Tower, Ho Sin Hang Campus
Abstract
Statistical learning allows us to detect hard-to-discern patterns from high dimensional, noisy and complex datasets. This capability is particularly well-suited to biomedical applications, especially cancer prognosis and prediction that depend on complex omics measurements. Hypervariability and latent heterogeneity that often exist in such datasets pose great challenge to traditional statistical learning methods, and can lead to inaccurate results. In this talk, we sample two methods from our work in statistical learning that meet this challenge. One is a new biclustering method that handles hypervariability by simultaneously dissecting mean and variance structures in the data. The other is a new classification method that accommodates latent heterogeneous subclasses by using composite classifiers. By applying the new methods to various high dimensional biomedical datasets, we demonstrate their strength in detecting cancer subtypes and predicting Alzheimer disease prognosis.
Biography
Guanhua is an Assistant Professor of Biostatistics and Medical Informatics at University of Wisconsin-Madison. He got his PhD from University of North Carolina at Chapel Hill in 2014 under the direction of Dr. Michael Kosorok. Before joining UW in 2017, he was an Assistant Professor of Biostatistics at Vanderbilt University. His research focuses on developing statistical learning methods for clinical and biomedical research. In particular, he is interested in analyzing heterogeneous, high-dimensional-omics data (genome, microbiome) and electronic health record data to advance precision medicine.